Research Article | Open Access
TWIST1/miR-199a axis promotes tumor aggressiveness through inhibiting oxidative phosphorylation in carcinomas
Umar Raza1, Debmalya Roy2
1Department of Biological Sciences, National University of Medical Sciences (NUMS), Abid Majeed Road, Rawalpindi, Pakistan.
2Institute of Pharmacy, Kalyani, India.
Correspondence: Umar Raza (Department of Biological Sciences, National University of Medical Sciences (NUMS), Abid Majeed Road, Rawalpindi, Pakistan; E-mail: umarraza698@gmail.com).
Asia-Pacific Journal of Surgical & Experimental Pathology 2024, 1: 81-93. https://doi.org/10.32948/ajsep.2024.11.26
Received: 06 Jun 2024 | Accepted: 28 Nov 2024 | Published online: 08 Dec 2024
Methods In this study, we employed a stringent pan-cancer multi-omic metabolism-targeted differential expression approach to identify the metabolic hubs regulating tumor aggressiveness. mRNA, miRNA, DNA methylation and mutation profiling data of tumors representing 14 different types of carcinomas was downloaded from TCGA database. Cell line expression profiling and drug response data was downloaded from CCLE database. Pathway enrichment, GSEA, String protein-protein interaction, miRNA-mRNA prediction, network random-walk and CCLE drug response analyses were carried out.
Results We identified downregulated expression of enzymes involved in oxidative phosphorylation as a key common factor across carcinomas, aligning with the Warburg effect. Additionally, we established that the decreased dependence on oxidative phosphorylation is driven by elevated expression of miR-199 family miRNAs that inhibit their expression at the post-transcriptional level. Furthermore, we identified the epithelial-to-mesenchymal transition-related transcription factor, TWIST1, as a master regulator of tumor aggressiveness by controlling miR-199a-3p and -5p expression. Random walk analysis of established miRNA-mRNA network identified NDUFA2, DLD, COX15, NDUFB5, and TIMM13 as crucial metabolic hubs downregulated as tumors become aggressive. Drug response analysis suggested that targeting PDGFR signaling may offer a novel therapeutic approach to counteract the aggressiveness driven by the loss of oxidative phosphorylation.
Conclusion We identified TWIST1/miR-199a axis mediated suppression of oxidative phosphorylation as major metabolic contributor towards tumor aggressiveness in carcinomas. These insights underscore the critical interplay between metabolic reprogramming and tumor aggressiveness, opening avenues for potential metabolic therapies in clinical settings.
Key words warburg effect, oxidative phosphorylation, miR-199a, TWIST1, PDGFR
The Cancer Genome Atlas (TCGA) (https://www.cancer.gov/tcga) offers multiple types of genomic and transcriptomic data including mutation, methylation, and protein coding and non-coding expression profiling data from large number of patients representing multiple different cancer type along with subset of matched normal tissues. This data has already been used to great extent in efficiently filling the important gaps in knowledge about cancer biology, particularly related to drivers of oncogenesis and immune response in cancers of different anatomical sites [9, 10]. For instance, researchers have devised novel ways to classify tumors by immune-profiling of cancer cells of different origin [11] or by survival outcome of patients [12]; thereby, helped in assigning better treatment course for chemoresistant patients [7]. Others have highlighted the potential of combination therapy by revisiting the oncogenic molecular pathways using pan-cancer approach [10]. Although these recent insights highlight the importance of using genomic and transcriptomic data to understand cancer associated molecular reprogramming and to device treatment course accordingly, they lack in identifying common metabolic vulnerabilities between cancers arising at different anatomical sites. Readily available genomic and transcriptomic data at TCGA database provides opportunity to explore the mechanisms cancer cells utilize to reprogram metabolic pathways in their favour and to investigate the crosstalk of reprogrammed metabolism with oncogenic pathways.
Involvement of genetic and epigenetic changes in controlling metabolic gene expression also poses a great challenge to understand cancer associated metabolic reprogramming. Temporal expression from a locus is under the control of multiple mechanisms throughout the cell. Changes in genomic methylation pattern may alter the gene expression through enhancing the recruitment of transcriptional repressors and/or by inhibiting the transcription factor (TF) binding at promoter [13]. On the other hand, perturbed mRNA stability due to post-transcriptional targeting by microRNAs (miRNAs) may also regulate gene expression. miRNAs are small 20-22 nucleotide non-coding RNAs which are transcribed from intergenic regions or by their own promoters. Over the years, miRNAs have been implicated in cancer development, progression, metastasis and therapy resistance [14]. The roles miRNAs play in wide range of cellular processes cannot be overlooked as 60% of human protein coding genes are predicted to be selectively regulated by miRNAs [15]. In line with this, multiple miRNAs have also been identified as key players of cancer cell metabolism by targeting enzymes involved in glucose transport, glycolysis, oxidative phosphorylation, and lactate and glutamine metabolism [16, 17]. These studies, while informative, lacks the assessment of genome wide perturbations in the expression of metabolic genes caused by miRNAs working in close conjunction.
Aggressive tumors generally do not respond well to therapeutic interventions aimed to target oncogenic signaling pathways. Therapy resistance is inevitable in almost all the cases due to multiple factors including acquisition of multi-drug resistance, inhibition of apoptotic cell death, hyperactivation of alternate growth mechanisms, alterations in drug metabolism and many more [18]. In comparison to therapies targeting oncogenic signaling mechanisms, metabolic therapies have provided an attractive approach in the clinic due to their evasion from drug resistance [19]. Therefore, the aim of this study was to comprehensively assess the metabolic reprogramming involved in tumor aggressiveness, and to identify the associated miRNA-mRNA network and metabolic hubs using a pan-cancer genome approach which can be therapeutically targeted to treat aggressive tumors in the clinic. In this line, we have found that gene expression of enzymes involved in oxidative phosphorylation gets successively downregulated as tumor become aggressive and identified that Twist Family BHLH Transcription Factor 1 (TWIST1) regulated miRNAs from miR-199 family work as upstream regulators of tumor aggressiveness by targeting genes involved in oxidative phosphorylation. Notably, changes in DNA methylation pattern were not found involved in tumor aggressiveness associated suppression of oxidative phosphorylation. Five genes namely NADH Oxidoreductase Subunit A2 (NDUFA2), Dihydrolipoamide Dehydrogenase (DLD), Cytochrome C Oxidase Assembly Homolog COX15 (COX15), NADH Oxidoreductase Subunit B5 (NDUFB5) and Translocase of Inner Mitochondrial Membrane 13 (TIMM13) which code for different enzymes involved in oxidative phosphorylation were found as metabolic hubs whose downregulations are associated with increased tumor aggressiveness. The results from this study also suggest that targeting Platelet-derived growth factor receptor (PDGFR) in aggressive tumors may inhibit loss of oxidative phosphorylation-driven tumor aggressiveness.
TCGA database encompasses mRNA, miRNA, DNA methylation and mutation profiling of more than 30 different cancer types with varying number of patients for each cancer type. In order to have statistically comparable results between different cancer types, only the cancer types having data from more than 300 patients were selected to be included in the analysis. In addition, cancer types arising in brain (Glioma and Glioblastoma) were excluded from the analysis because of the reasons that 1) tumors developed in these tissues are relatively distant from those arising in epithelial tissue in terms of tumor onset, growth and progression and 2) these tumors generally spread to adjacent brain tissues but do not metastasize to distant organs. This way, cancer patients’ Gene expression, DNA methylation and miRNA expression data representing 14 different cancer types (Bladder urothelial Carcinoma (BLCA), Breast invasive Carcinoma (BRCA), Colon Adenocarcinoma (COAD), Head and Neck Squamous cell Carcinoma (HNSC), Kidney Renal Clear cell carcinoma (KIRC), Kidney Renal Papillary cell carcinoma (KIRP) Liver Hepatocellular Carcinoma (LIHC), Lung Adenocarcinoma (LUAD), Lung Squamous cell Carcinoma (LUSC), Ovarian serous Carcinoma (OVCA), Prostate Adenocarcinoma (PRAD), Stomach Adenocarcinoma (STAD), Thyroid Carcinoma (THCA) and Uterine Corpus Endometrial Carcinoma (UCEC)) at TCGA database were retrieved online from Broad GDAC Firehose: https://gdac.broadinstitute.org/. Gene mutation data for different cancer types was retrieved from cBioportal for Cancer Genomics: https://www.cbioportal.org/. Gene lists associated with metabolic pathways were retrieved from KEGG database: https://www.genome.jp/kegg/pathway.html and Mammalian Metabolic Enzyme Database: https://hpcwebapps.cit.nih.gov/ESBL/Database/MetabolicEnzymes/MetabolicEnzymeDatabase.html, and were combined to develop metabolic gene signature of 2260 genes. Gene Expression data for cancer cell lines representing different cancer types was retrieved from Broad Institute Cancer Cell Line Encyclopedia (CCLE) https://portals.broadinstitute.org/ccle. Drug response data for cancer cell lines was retrieved from Genomics of Drug Sensitivity in Cancer: https://www.cancerrxgene.org/.
Patients’ tumor aggressiveness classification
Clinical data of pathological tumor stage and pathological metastatic stage was not available for all the patients and for all the cancer types selected for analysis. That’s why, tumor tissues were planned to be classified into low, intermediate and high aggressiveness groups using gene signature based classification. Epithelial-to-mesenchymal transition (EMT) has been established as prime marker for tumor aggressiveness and metastasis [20-23]. We downloaded Hallmark_EMT gene signature from Gene Set Enrichment Analysis (GSEA) portal https://www.gsea-msigdb.org/ and calculated Z-scores of all the 200 genes in this signature for each patient in all the cancer types under study. Later, Z-scores of these 200 genes for each patients were summed to calculate the tumor aggressiveness score for each patient. Lastly, in each cancer type, patients were sorted according to their tumor aggressiveness score and were divided into three equal groups; low, intermediate and high.
Identification of differentially expressed metabolic genes (DEMGs), differentially methylated metabolic genes (DMMGs) and differentially expressed miRNAs (DEmiRs) associated with tumor aggressiveness
mRNA expression of genes listed in metabolic gene signature were compared among tumor aggressiveness groups using one way ANOVA to identify tumor aggressiveness associated DEMGs for each cancer type under study. DNA methylation of genes listed in metabolic gene signature were compared among tumor aggressiveness groups using one way ANOVA to identify tumor aggressiveness associated DMMGs in each cancer type under study. Expression of all miRNAs were compared among tumor aggressiveness groups using one way ANOVA to identify tumor aggressiveness associated DEmiRs for each cancer type under study. Significance cutoff of p-value < 0.05 was used in these analyses.
Gene expression, gene methylation, miRNA expression and gene signature scores
In order to calculate gene expression scores associated with tumor aggressiveness, first successive change in expression of metabolic genes from low to high tumor aggressiveness groups was identified. If a gene was successively upregulated from low to high tumor aggressiveness group, an aggressiveness score of 1 was given whereas if a gene was successively downregulated from low to high tumor aggressiveness group, an aggressiveness score of a -1 was given. All other genes showing haphazard changes from low to high tumor aggressiveness group were given a score of 0. p-values less than 10-4 were transformed into 10-4. Later, if ANOVA p-value of a gene was less than 0.05, its aggressiveness score was multiplied with –log10(p-value) to identify its tumor aggressiveness associated expression score, otherwise, it was multiplied with 0 to exclude the metabolic genes not significantly differentially expressed among tumor aggressiveness groups. In a similar way, tumor aggressiveness associated metabolic gene methylation and miRNA expression scores were calculated. To calculate miRNA-methylation scores, methylation value of two genes (MIR199A1 and MIR199A2) was averaged for each patient. Gene signature scores for each patient or cell line were calculated by summing the Z-scores of all the genes involved in that signature.
Pathway enrichment analysis
Pathway enrichment analysis was performed using online freely available DAVID functional annotation tool: https://david.ncifcrf.gov/summary.jsp. Briefly, 1) list of DEMGs from each cancer type, 2) list of DEMGs common to more than 7 cancer types, 3) genes whose expression was negatively correlated with their methylation individually in individual cancer type, 4) genes whose expression was negatively correlated with their methylation in more than 7 cancer types, and 5) genes whose expression was negatively correlated with 6 selected miRNAs in more than 7 cancer types were uploaded to DAVID platform to identify the associated KEGG pathways. Significance cutoff of False Discovery Rate (FDR) < 0.05 was used.
miRNA-mRNA target prediction
miRNA-mRNA target prediction data was retrieved from miRDIP4 tool: http://ophid.utoronto.ca/mirDIP/. Briefly, list of oxidative phosphorylation associated DEMGs enriched in more 7 cancer types was uploaded to miRDIP4 tool along with miR-199a-3p, miR-199a-5p and miR-199b-3p. The tool predicted a total of 58 interactions between these 3 miRNAs and 40 genes with high confidence.
Protein-protein interactions
List of genes predicted as targets of miR-199a-3p and miR-199a-5p was uploaded to the Search Tool for the Retrieval of Interacting Genes (STRING) database: https://string-db.org. Confidence threshold 0.4 was used to identify the protein-protein interactions between the predicted targets of miRNAs.
Network construction and identification of tumor aggressiveness associated metabolic hubs
All the miRNA-mRNA interactions and protein-protein interactions were combined into one file. Interaction file was then uploaded to Cytoscape software v3.7.1 to construct tumor aggressiveness associated miRNA-mRNA network. In Cytoscape, node size was modified to express the number of cancer types in which a specific gene/node is associated with tumor aggressiveness whereas edge girth was modified to show the confidence score for miRNA-mRNA or mRNA-mRNA interactions. In order to identify the hub genes in the network, energy driven random walk analysis with restart was performed. Briefly, for each cancer type, a constant energy was introduced into the network through the driver nodes (TWIST1, miR-199a-3p and miR-199a-5p) and random walk analysis with restart was performed for N number of iterations until energy got distributed into the network and energy flow came to a steady state. Later, energy retained by miRNA targets was ranked for each cancer type and these ranks were averaged across cancer types for each gene. Tumor aggressiveness associated metabolic hubs were identified by calculating 1/average Rank score.
CCLE drug response analysis
Firstly, 329 cell lines representing 15 different cancer types were selected from the CCLE database based on the availability of their drug response data at Genomics of Drug Sensitivity in Cancer database. Hallmark_EMT, TWIST1 and OxPhos-sig scores were calculated for all the cell lines. Like patient tumors, these cell lines were also classified into low, intermediate and high aggressiveness groups, and TWIST1 expression and OxPhos-sig scores were compared. Later, top 25 cell lines having low tumor aggressiveness score and high OxPhos-sig score (Group 1), and top 25 cell lines having high tumor aggressiveness score and low OxPhos-sig score (Group 2) were identified. In order to identify top targeted therapies to which cell lines in Group 2 were sensitive whereas cell lines in Group 1 were resistant, a correlation based method was used. Briefly, targeted therapies whose response (sensitivity to resistance) was positively correlated with tumor aggressiveness score (R > 0.30) and negatively correlated with oxidative phosphorylation score (R < -0.30) were selected for further analysis. In addition, gene expression of targets of these therapies was checked in TCGA database to identify the genes targeting which may have potential to suppress the tumor aggressiveness.
Statistical analysis
One way ANOVA was used to compare more than two groups during identification of DEMGs, DMMGs and DEmiRs associated with tumor aggressiveness. Correlation between miRNA and gene expression, between miRNA methylation and expression, between gene methylation and expression, between transcription factor (TF) expression and miRNA expression, and others were calculated using Pearson correlation coefficient. Significance cutoff of p-value < 0.05 was used unless otherwise stated.
In order to identify the metabolic pathways associated with tumor aggressiveness, we downloaded the gene expression data of tumor tissues representing 14 different cancer types from TCGA database (Figure 1A) and divided the patients into having low, intermediate or highly aggressive tumors based on their EMT signature scores (Figure 1B; For Details, see Materials and Methods Section). Next, we compared the changes in expression of genes involved in metabolic pathways (For Details, see Materials and Methods Section) among tumor aggressiveness groups (Low, Intermediate, High) and identified the tumor aggressiveness associated differentially expression metabolic genes (DEMGs) for each cancer type separately (Figure 1C and D). Later, we performed KEGG pathway enrichment analyses using lists these DEMGs from each cancer type and identified oxidative phosphorylation as top dysregulated pathway among all the cancer types (Figure 1E). In addition, we also performed KEGG pathway enrichment analysis using list of tumor aggressiveness associated DEMGs common to more than 7 cancer types and again found oxidative phosphorylation as top dysregulated pathway associated with tumor aggressiveness (Figure 1F). Next, we calculated the expression score of genes involved in regulation of oxidative phosphorylation (For Details, See Materials and Methods Section) and found that most of the these genes get successively downregulated as tumor becomes aggressive (Figure 1G) confirming that tumor cells tend to suppress oxidative phosphorylation to become aggressive.
DNA methylation do not regulate tumor aggressiveness associated suppression of oxidative phosphorylation
Changes in DNA methylation pattern can alter the gene expression by enhanced recruitment of transcriptional repressors and/or by inhibition the TF binding [13]. In order to identify a general mechanism involved in suppression of oxidative phosphorylation, we aimed to check whether overall changes in DNA methylation pattern affect oxidative phosphorylation during tumor cells’ shift towards more aggressive phenotype. In this line, we downloaded the DNA methylation data of tumor tissues representing 14 different cancer types from TCGA database (Figure 1A). Then, we compared the changes in DNA methylation of genes involved in metabolic pathways (For Details, see Materials and Methods Section) among tumor aggressiveness groups (Low, Intermediate, High) and identified varying number of differentially methylated metabolic genes (DMMGs) associated with tumor aggressiveness for each cancer type (Figure 2A and B) suggesting that changes in DNA methylation may affect metabolic processes and help tumors in becoming aggressive. As DNA methylation is generally inversely correlated with gene expression, we also checked the correlation between DNA methylation and gene expression of metabolic genes and for each cancer type, found higher number of genes whose expression was negatively correlated with their DNA methylation compared to number of genes whose expression was positively correlated with their DNA methylation (Figure 2C) again suggesting that DNA methylation may play important role in tumor aggressiveness. Next, we performed KEGG pathway enrichment analyses separate for each cancer type using lists of genes whose expression was negatively correlated with their DNA methylation status (threshold R ≤ -0.2) (Figure 2D) and combined using list of genes whose expression was negatively correlated with their DNA methylation status (threshold R ≤ -0.2) in more than 7 cancer types (Figure 2E). Although changes in methylation pattern of different metabolic pathways was found associated with tumor aggressiveness, oxidative phosphorylation did not come up among top hits (Figure 2D and E) suggesting that DNA methylation does not affect genes involved in oxidative phosphorylation during tumor aggressiveness.
miR-199 family may regulate oxidative phosphorylation and promote tumor aggressiveness
Next, in order to identify non-coding players of tumor aggressiveness, we downloaded the miRNA expression data of tumor tissues representing 14 different cancer types from TCGA database (Figure 1A). we compared the changes in miRNA expression (For Details, see Materials and Methods Section) among tumor aggressiveness groups (Low, Intermediate, High) and identified varying number of differentially expressed miRNAs (DEmiRs) associated with tumor aggressiveness for each cancer type (Figure 3A and B). Six miRNAs were found consistently differentially expressed among tumor aggressiveness groups in all the 14 cancer types. As miRNAs lead to inhibition of their target mRNAs and alter their expression to produce measurable effects [14], we was interested in identifying the functional mRNA targets of these 6 miRNAs. To this end, using a genome-wide approach, we calculated the correlation between expression of these 6 miRNAs and mRNA expression from all the protein coding genes (rather than those from metabolic genes), and selected the genes negatively correlated with individually to each of these miRNAs in > 7 cancer types. Later, we performed KEGG pathway enrichment analysis to identify the potential pathways in which these miRNAs may be involved. Interestingly, negatively correlated genes with 3 of these miRNAs were enriched in oxidative phosphorylation (Figure 3C). These 3 miRNAs (miR-199a-3p, miR-199a-5p and miR-199b-3p) belong to miR-199 family and their expression was also found to be gradually increasing between low and high tumor aggressiveness groups in all 14 different cancer types (Figure 3D).
TWIST1 may suppress oxidative phosphorylation by directly promoting miR-199a-3p and miR-199a-5p expression
Next using miRDIP4 online tool, we predicted the targets of three miRNAs associated with tumor aggressiveness among oxidative phosphorylation associated genes. Forty genes associated with oxidative phosphorylation were identified as direct targets of these three miRNAs with high confidence level. Interestingly, all the targets of miR-199b-3p were redundant in targets of miR-199a-3p and miR-199a-5p, that’s why we excluded miR-199b-3p from further analysis (Figure 4A). Next, we was curious that how these two shortlisted tumor aggressiveness associated miRNA are regulated during tumor cell transition towards more aggressive phenotype. Interestingly, literature suggests that two loci in the human genome, MIR199A1 on chromosome 19 and MIR199A2 on chromosome 1, encode the precursors of miR-199a-3p and miR-199a-5p under the control of DNA methylation and/or transcription factors Twist Family BHLH Transcription Factor 1 (TWIST1) and Early Growth Response 1 (EGR1) [24, 25]. TWIST1 is already established as one of the major regulators of EMT and tumor aggressiveness [26] whereas DNA methylation can alter the gene expression pattern in multiple ways including recruitment of transcriptional repressors and/or by inhibiting the TF binding [13]. Based on these, we hypothesized that TWIST1 may regulate the expression of these miRNAs during tumor aggressiveness with the help of DNA methylation. In order to address this, we checked the DNA methylation status of MIR199A1 and MIR199A2 genes and found that these two loci were successively hypomethylated during tumor aggressiveness in almost all the cancer types (Figure 4B). In addition, we also found negative correlation between methylation and miRNA expression from these two loci suggesting that methylation does effect the expression of these miRNAs (Figure 4C). Next, we also checked the correlation between expression of TFs (TWIST1 and EGR1) and miRNAs and found that expression of TWIST1, but not of EGR1, was almost always positively correlated with the expression of these miRNAs (Figure 4D) supporting that TWIST1 mediated regulation of these miRNAs could be a mechanism through which cancer cell gradually suppress oxidative phosphorylation as they become aggressive. Notably, we could not find any specific pattern of DNA methylation (except for miRNAs) or gene mutations associated with targets we identified for miR-199a-3p and miR-199a-5p (Figure 5A and B). Next, we developed TF-miRNA-mRNA network entailing 1 TF, 2 miRNAs and their 40 targets (Figure 4E) and performed RandomWalk with restart algorithm to identify molecular hubs in the network [27]. As a result, 5 genes namely NDUFA2, DLD, COX15, NDUFB5 and TIMM13 as the most important metabolic hubs. These results suggest that restoring oxidative phosphorylation or modulating identified metabolic hubs through fine tuning TWIST1/miR-199a via therapeutic targeting at clinical level may help in treating aggressive tumors efficiently.
Targeting PDGFR signaling may inhibit tumor aggressiveness
TWSIT1 has been reported to be not much expressed in healthy tissue but has been found upregulated in multiple cancer types. Unfortunately, not a single approved therapeutic treatment is available to target TWIST1 in human cancers [28]. In addition, miRNA and miRNA inhibitor based therapies are still underdeveloped [29]. In a quest to find a therapeutic option for patients having aggressive tumors based on the findings of this project, we analyzed online available drug response data of different cancer cell lines. Not surprisingly, cancer cell lines also showed a pattern of increased TWIST1 expression and decreased oxidative phosphorylation between low to highly aggressive cell lines (Figure 6A-D). Next, we compared the drug response of top cell lines having low tumor aggressiveness score and high OxPhos-sig score (G1) with those having high tumor aggressiveness score and low OxPhos-sig score (G2) and identified multiple therapeutic agents to which cancer cell lines in G2 showed sensitivity (Figure 6E). we checked the expression of gene targets of identified drugs in TCGA database (Figure 6F) and found 2 genes expressing subunits of PDGFR (PDGFRA and PDGFRB) that drive PDGFR signaling (Figure 6F). Genes expressing platelet-derived growth factor (PDGFA-D) were also found successively upregulated to certain extent as tumors become aggressive (Figure 6G).
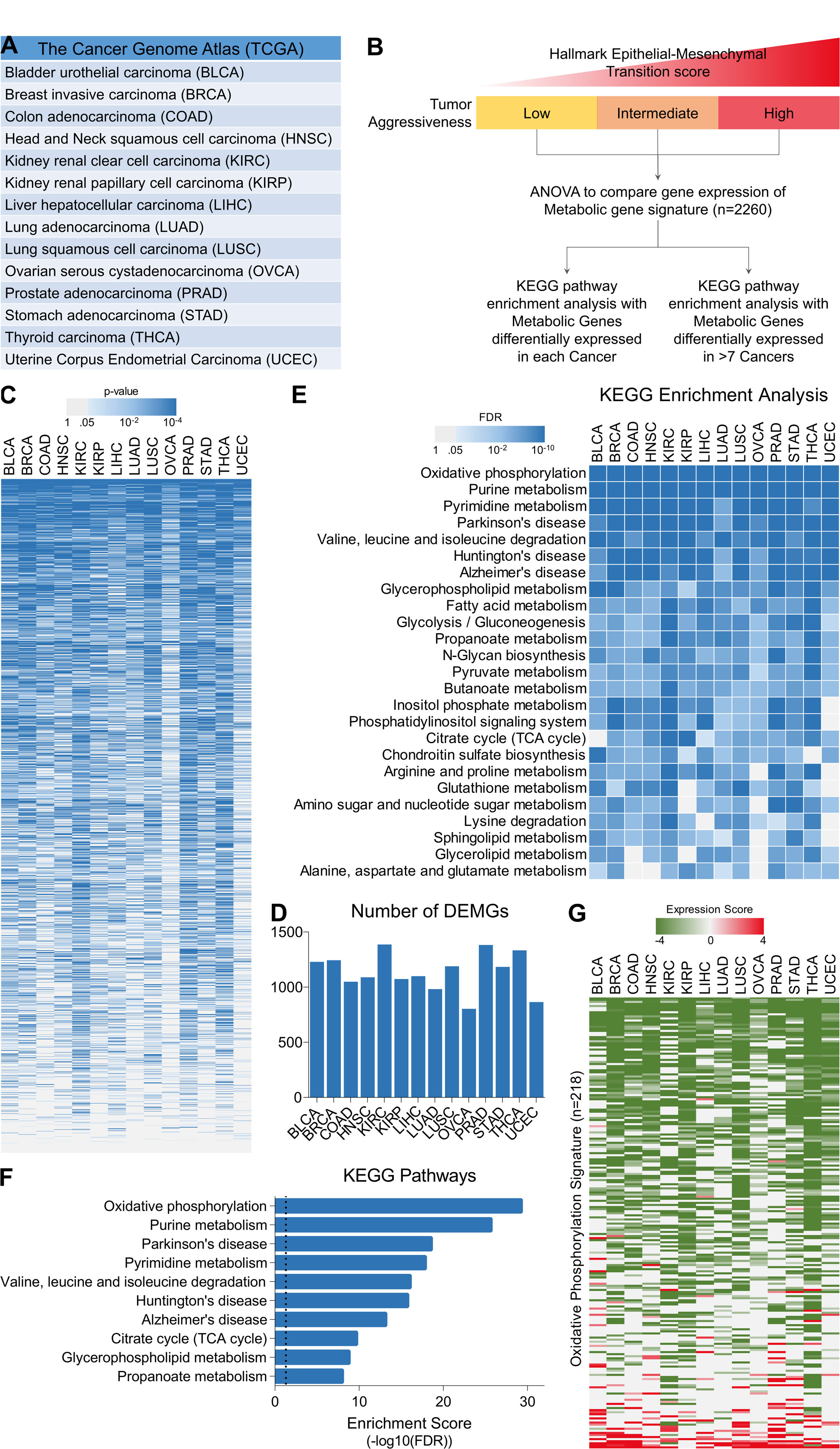
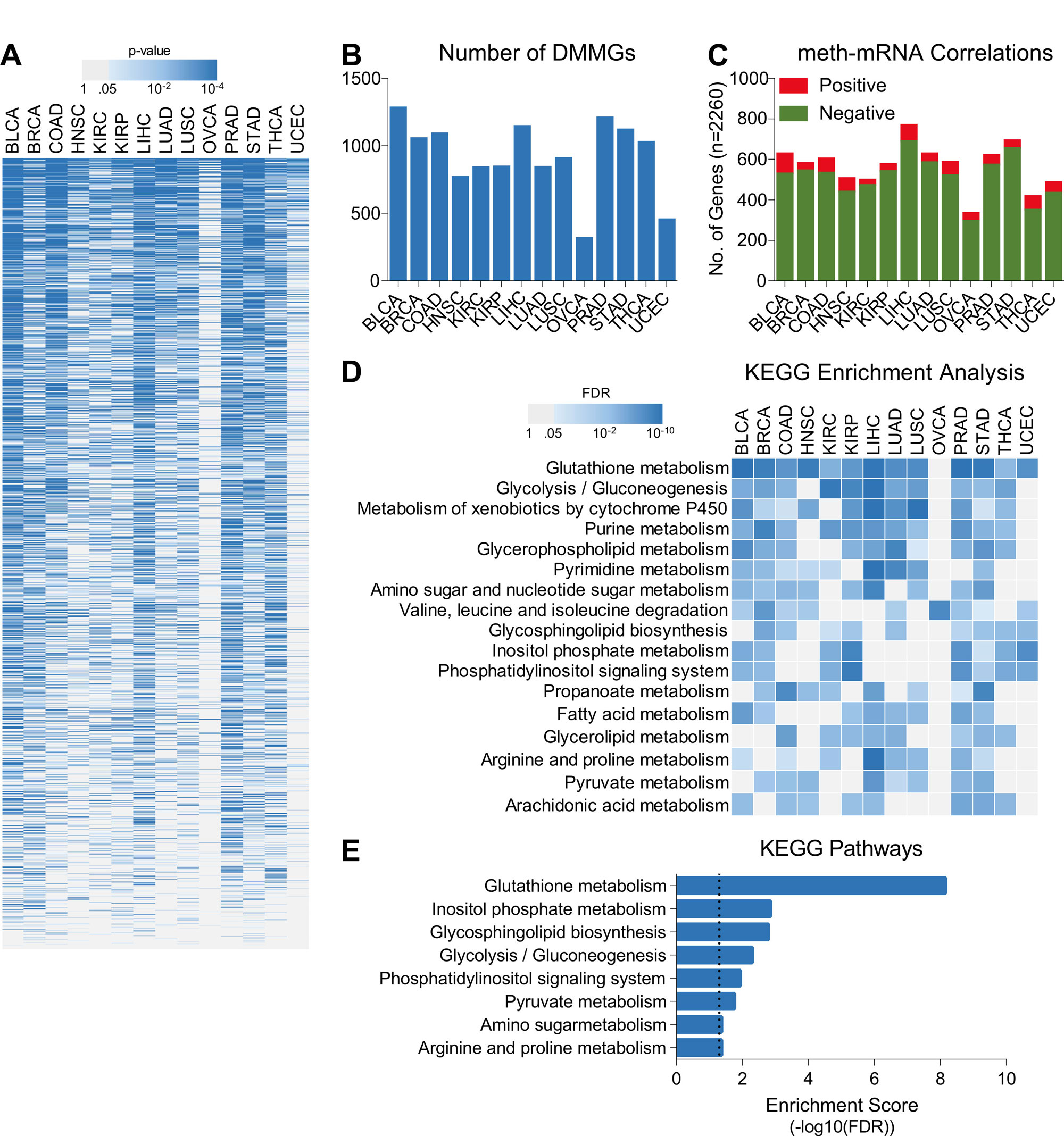
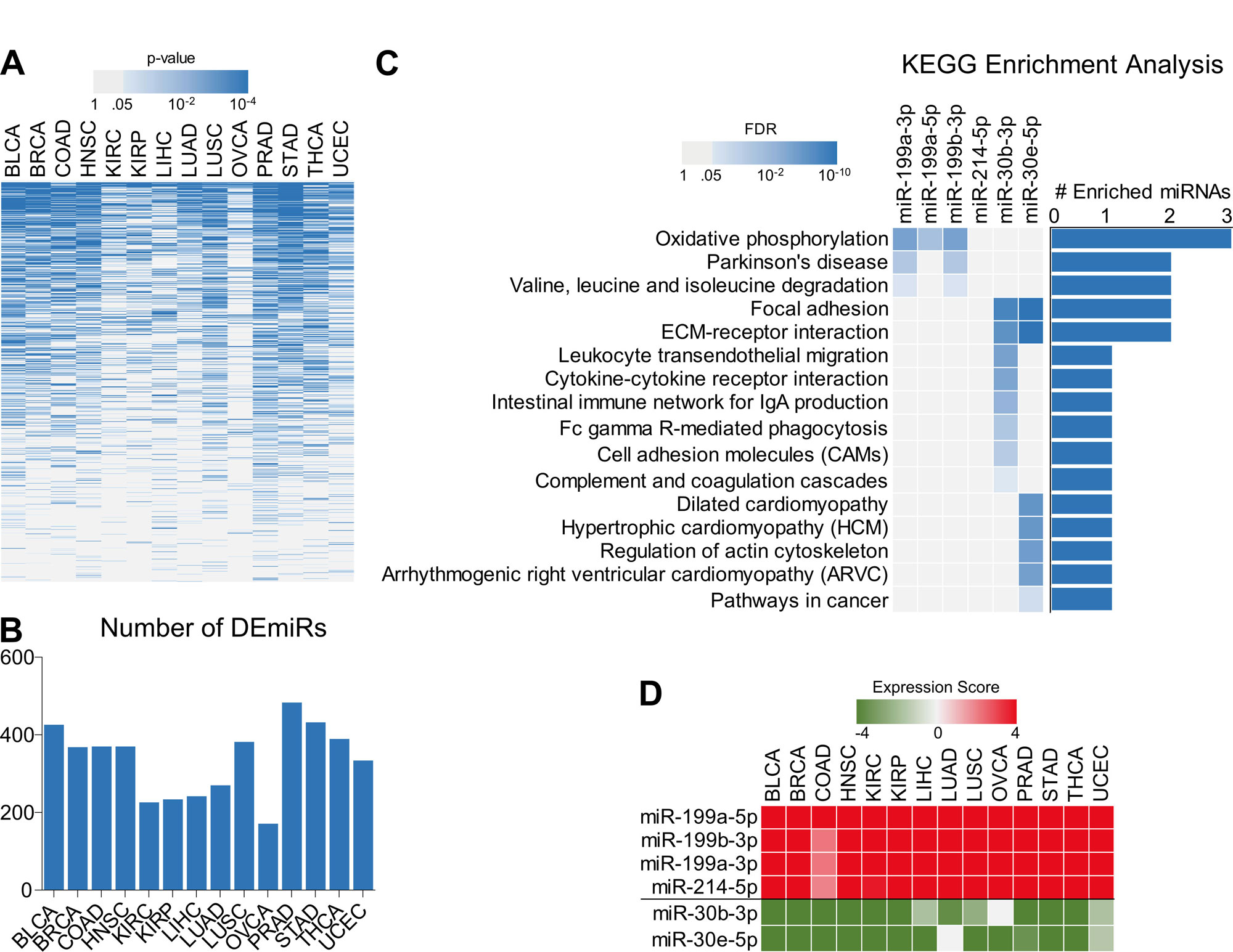
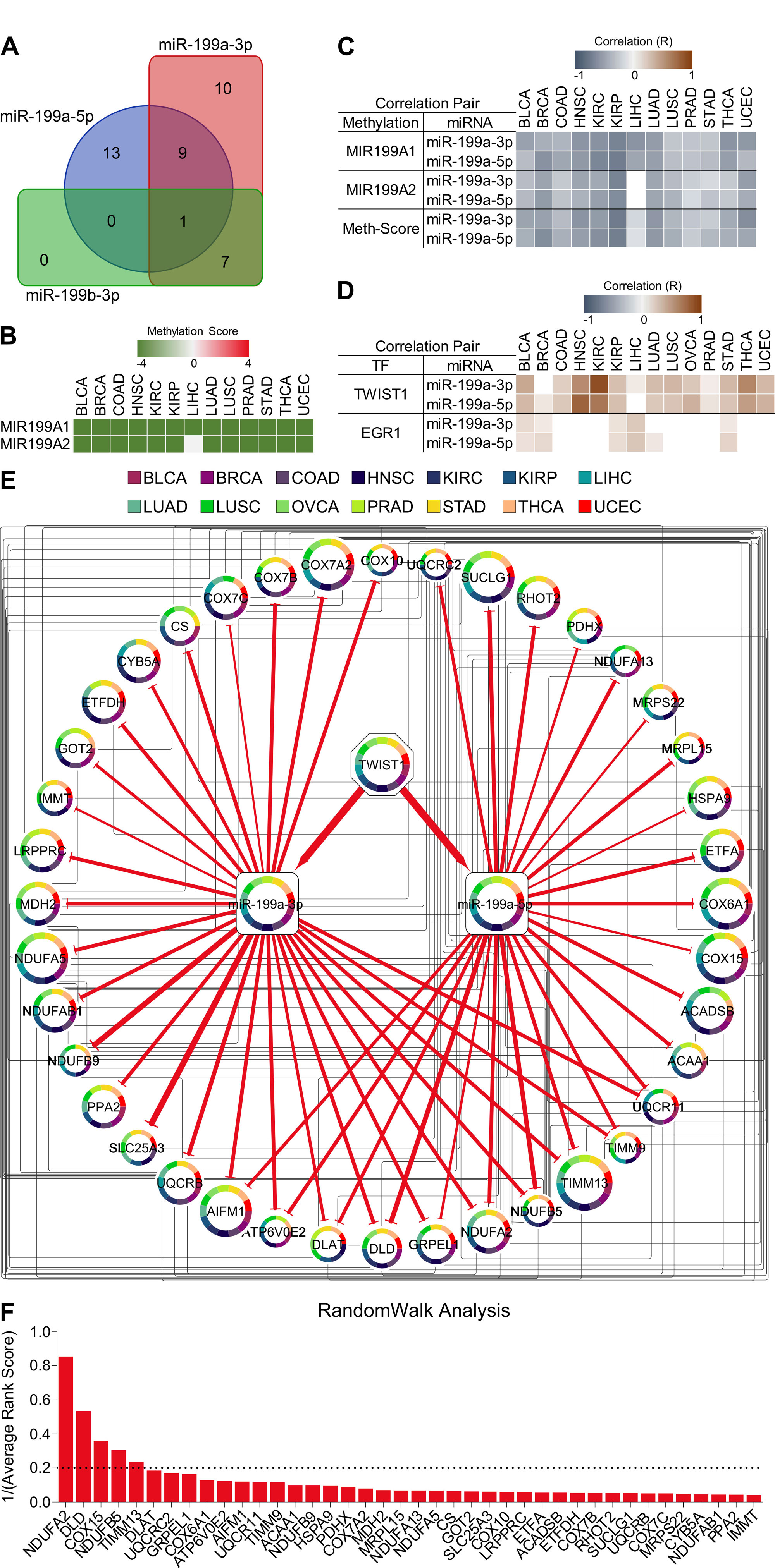
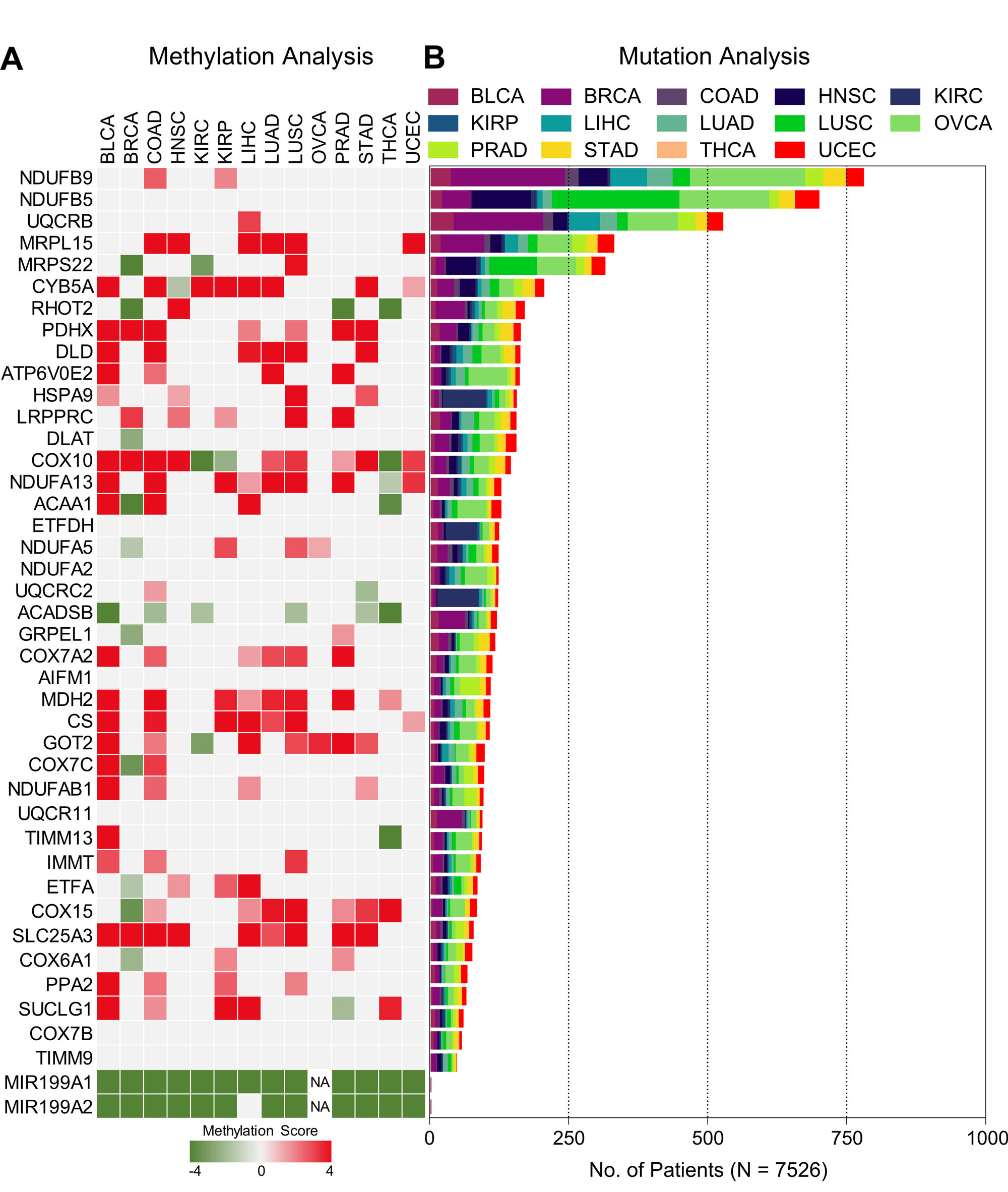
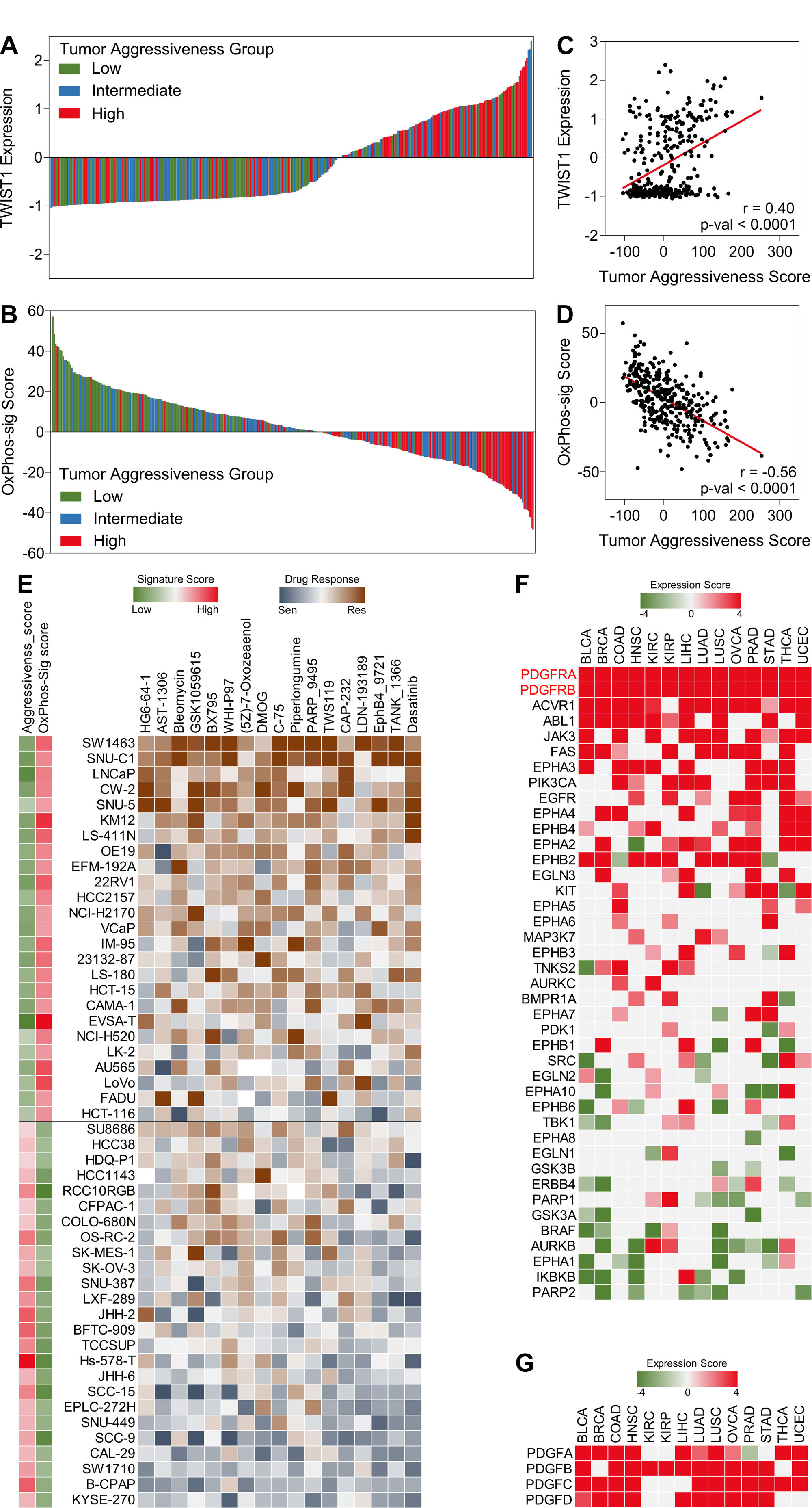
Over the years, varying tumor promoting and limiting roles have been discovered for miR-199a family miRNAs depending upon the cancer tissue they express in and the mRNAs they target under specific conditions [24, 32]. For instance, miR-199a-3p has the potential to inhibit hepatocellular carcinoma (HCC) growth by targeting oncogenic factors such as mammalian target of rapamycin (mTOR), tyrosine-protein kinase Met (c-met), and p21 (RAC1) activated kinase 4 (PAK4) [33]. On the other hand, miR-199a-3p suppresses zinc fingers and homeoboxes 1 (ZHX1) expression by binding to its 3ʹUTR, leading to the enhancement of gastric cancer cell growth and proliferation [34]. Similarly, miR-199a-5p has been found downregulated in lung and colorectal cancers compared to respective normal tissues and has been reported to inhibit proliferation in these cancer types by targeting hypoxia inducible factor alpha (HIF1A) [35, 36]. On the other hand, miR-199a-5p was found upregulated in gastric cancer where it inhibited apoptosis by targeting SMAD4 [32]. The results from the in silico analyses performed in this study suggest a tumor aggressiveness promoting role for these miR-199 family miRNAs (Figure 3); thus, aligning with and expanding on the known roles of these miRNAs in cancer, which need to be further validated in vitro and in vivo.
TWIST1 is already established as one of the major regulators of EMT and tumor aggressiveness [26] which has also been shown to regulate miR-199 family miRNAs at transcriptional level [37]. We identified a consistent downregulation of oxidative phosphorylation associated genes in aggressive tumors along with upregulation miR-199a miRNAs at the downstream of TWIST1 (Figure 1 and 4). The downregulation of oxidative phosphorylation-related genes is regulated primarily by miRNAs rather than DNA methylation. This is evidenced by the significant inverse correlation between the expression of miR-199a-3p/miR-199a-5p and OXPHOS genes, and the lack of significant changes in DNA methylation patterns affecting these genes (Figure 2 and 4). This miRNA-mediated regulation underscores the importance of post-transcriptional control in cancer metabolism [38, 39]. Random walk analysis is a computational method used in network biology to identify hub genes or important nodes within a biological network [40]. Through random walk analysis on our miRNA-mRNA network, we identified NDUFA2, DLD, COX15, NDUFB5 and TIMM13 as metabolic hubs crucial to maintain metabolic harmony by ensuring oxidative phosphorylation (Figure 4). These genes are critical components of the mitochondrial respiratory chain and their suppression is associated with increased tumor aggressiveness. NDUFA2, NDUFB5, and COX15 are involved in electron transfer within the respiratory chain, contributing to the generation of ATP, and their dysregulation has been implicated in cancer [41, 42]. DLD is a key enzyme in the pyruvate dehydrogenase complex, connecting glycolysis to the citric acid cycle and ultimately feeding electrons into the respiratory chain. It has been found downregulated in other cancer types as well [43]. TIMM13 is part of the mitochondrial import machinery, facilitating the translocation of proteins into the mitochondria for proper assembly and function of respiratory chain complexes. Although recent finding have suggested a tumor promoting role for TIMM13 [44], our findings highlight a tumor suppressive role for this gene (Figure 4).
Given the regulatory role of miR-199 family miRNAs in suppressing OXPHOS and promoting tumor aggressiveness, targeting these miRNAs or their downstream pathways could be a promising therapeutic strategy. However, direct miRNA-targeted therapies are still in their early stages of development [29]. Therefore, alternative approaches, such as targeting upstream regulator TWIST1 offers a more feasible opportunity in the near term. However, there are currently no approved therapies targeting TWIST1 directly [28]. Therefore, we employed a drug response analysis approach to identify the drugs which can best suit to inhibit loss of oxidative phosphorylation-driven tumor aggressiveness in cancer and suggest that targeting PDGFR signaling, may be effective in this scenario (Figure 6). PDGFR mediated signaling networks have long been implicated in different cancer associated mechanisms including oncogenesis, tumor progression and metastasis [45, 46]. The question arises that whether PDGFR signaling is an upstream regulator of TWIST1 or a downstream player that gets hyperactivated in response to metabolic shift. Notably, both notions have been previously proven in independent studies. For instance, PDGF has been shown to promote tumor aggressiveness by upregulating TWIST1 in a notch dependent manner [47], whereas TWIST1 mediated upregulation of PDGFRs has been implicated in metastasis and cancer stemness [48, 49].
Our study's findings, along with the observation that cells with low oxidative phosphorylation levels are sensitive to treatments targeting PDGFR signaling (Figure 6), highlight the potential value of investigating therapeutic interventions focused on PDGFR signaling. These investigations, both in vitro and in vivo in cancer cells, could pave the way for developing revised treatment options for patients with aggressive diseases. Notably, dasatinib, an FDA-approved tyrosine kinase inhibitor targeting PDGFR, SRC, and BCR-ABL, has shown promise in treating various cancers [50]. In our analysis, aggressive cancer cell lines with high TWIST1 expression and low oxidative phosphorylation were sensitive to dasatinib (Figure 6). By inhibiting PDGFR signaling, dasatinib may disrupt the metabolic shifts that sustain tumor aggressiveness, offering a promising approach for treating aggressive cancers characterized by low oxidative phosphorylation activity. Additionally, it is crucial to test other PDGFR inhibitors to validate and potentially translate our findings into clinical applications.
No applicable.
Ethics approval
No applicable.
Data availability
The data will be available upon request.
Funding
This project was funded by the Higher Education Commission of Pakistan (SRGP # 21-2075) awarded to Dr. Umar Raza.
Authors’ contribution
UR performed data analyses. UR drafted the manuscript.
UR and DR prepared figures and graphical representations. UR
and DR critically revised the manuscript. All authors approved the final version of manuscript.
Competing interests
None.
- Warburg O, Wind F, Negelein E: THE METABOLISM OF TUMORS IN THE BODY. J Gen Physiol 1927, 8(6): 519-530.
- Dang CV: Links between metabolism and cancer. Genes Dev 2012, 26(9): 877-890.
- Liu Y: Fatty acid oxidation is a dominant bioenergetic pathway in prostate cancer. Prostate Cancer Prostatic Dis 2006, 9(3): 230-234.
- Hanahan D, Weinberg RA: Hallmarks of cancer: the next generation. Cell 2011, 144(5): 646-674.
- Nilsson R, Jain M, Madhusudhan N, Sheppard NG, Strittmatter L, Kampf C, Huang J, Asplund A, Mootha VK: Metabolic enzyme expression highlights a key role for MTHFD2 and the mitochondrial folate pathway in cancer. Nat Commun 2014, 5: 3128.
- Hakimi AA, Reznik E, Lee CH, Creighton CJ, Brannon AR, Luna A, Aksoy BA, Liu EM, Shen R, Lee W, et al: An Integrated Metabolic Atlas of Clear Cell Renal Cell Carcinoma. Cancer Cell 2016, 29(1): 104-116.
- Wang F, Chang JT, Zhang Z, Morrison G, Nath A, Bhutra S, Huang RS: Discovering drugs to overcome chemoresistance in ovarian cancers based on the cancer genome atlas tumor transcriptome profile. Oncotarget 2017, 8(70): 115102-115113.
- Rosario SR, Long MD, Affronti HC, Rowsam AM, Eng KH, Smiraglia DJ: Pan-cancer analysis of transcriptional metabolic dysregulation using The Cancer Genome Atlas. Nat Commun 2018, 9(1): 5330.
- Berger AC, Korkut A, Kanchi RS, Hegde AM, Lenoir W, Liu W, Liu Y, Fan H, Shen H, Ravikumar V, et al: A Comprehensive Pan-Cancer Molecular Study of Gynecologic and Breast Cancers. Cancer Cell 2018, 33(4): 690-705.e9.
- Sanchez-Vega F, Mina M, Armenia J, Chatila WK, Luna A, La KC, Dimitriadoy S, Liu DL, Kantheti HS, Saghafinia S, et al: Oncogenic Signaling Pathways in The Cancer Genome Atlas. Cell 2018, 173(2): 321-337.e10.
- Hoadley KA, Yau C, Hinoue T, Wolf DM, Lazar AJ, Drill E, Shen R, Taylor AM, Cherniack AD, Thorsson V, et al: Cell-of-Origin Patterns Dominate the Molecular Classification of 10,000 Tumors from 33 Types of Cancer. Cell 2018, 173(2): 291-304.e6.
- Liu J, Lichtenberg T, Hoadley KA, Poisson LM, Lazar AJ, Cherniack AD, Kovatich AJ, Benz CC, Levine DA, Lee AV, et al: An Integrated TCGA Pan-Cancer Clinical Data Resource to Drive High-Quality Survival Outcome Analytics. Cell 2018, 173(2): 400-416.e11.
- Moore LD, Le T, Fan G: DNA methylation and its basic function. Neuropsychopharmacology 2013, 38(1): 23-38.
- Raza U, Zhang JD, Sahin O: MicroRNAs: master regulators of drug resistance, stemness, and metastasis. J Mol Med (Berl) 2014, 92(4): 321-336.
- Friedman RC, Farh KK, Burge CB, Bartel DP: Most mammalian mRNAs are conserved targets of microRNAs. Genome Res 2009, 19(1): 92-105.
- Pedroza-Torres A, Romero-Córdoba SL, Justo-Garrido M, Salido-Guadarrama I, Rodríguez-Bautista R, Montaño S, Muñiz-Mendoza R, Arriaga-Canon C, Fragoso-Ontiveros V, Álvarez-Gómez RM, et al: MicroRNAs in Tumor Cell Metabolism: Roles and Therapeutic Opportunities. Front Oncol 2019, 9: 1404.
- Ye J, Zou M, Li P, Liu H: MicroRNA Regulation of Energy Metabolism to Induce Chemoresistance in Cancers. Technol Cancer Res Treat 2018, 17: 1533033818805997.
- Mansoori B, Mohammadi A, Davudian S, Shirjang S, Baradaran B: The Different Mechanisms of Cancer Drug Resistance: A Brief Review. Adv Pharm Bull 2017, 7(3): 339-348.
- Liu Q, Tong D, Liu G, Xu J, Do K, Geary K, Zhang D, Zhang J, Zhang Y, Li Y, et al: Metformin reverses prostate cancer resistance to enzalutamide by targeting TGF-β1/STAT3 axis-regulated EMT. Cell Death Dis 2017, 8(8): e3007.
- Andergassen U, Schlenk K, Jeschke U, Sommer H, Kölbl A: Epithelial‑mesenchymal transition was identified as a potential marker for breast cancer aggressiveness using reverse transcription‑quantitative polymerase chain reaction. Mol Med Rep 2018, 18(2): 1733-1739.
- Sethi S, Sarkar FH, Ahmed Q, Bandyopadhyay S, Nahleh ZA, Semaan A, Sakr W, Munkarah A, Ali-Fehmi R: Molecular markers of epithelial-to-mesenchymal transition are associated with tumor aggressiveness in breast carcinoma. Transl Oncol 2011, 4(4): 222-226.
- Voulgari A, Pintzas A: Epithelial-mesenchymal transition in cancer metastasis: mechanisms, markers and strategies to overcome drug resistance in the clinic. Biochim Biophys Acta 2009, 1796(2): 75-90.
- Yang J, Weinberg RA: Epithelial-mesenchymal transition: at the crossroads of development and tumor metastasis. Dev Cell 2008, 14(6): 818-829.
- Gu S, Chan WY: Flexible and versatile as a chameleon-sophisticated functions of microRNA-199a. Int J Mol Sci 2012, 13(7): 8449-8466.
- Lee YB, Bantounas I, Lee DY, Phylactou L, Caldwell MA, Uney JB: Twist-1 regulates the miR-199a/214 cluster during development. Nucleic Acids Res 2009, 37(1): 123-128.
- Lu W, Kang Y: Epithelial-Mesenchymal Plasticity in Cancer Progression and Metastasis. Dev Cell 2019, 49(3): 361-374.
- Luo J, Liang S: Prioritization of potential candidate disease genes by topological similarity of protein-protein interaction network and phenotype data. J Biomed Inform 2015, 53: 229-236.
- Pei H, Li Y, Liu M, Chen Y: Targeting Twist expression with small molecules. Medchemcomm 2017, 8(2): 268-275.
- Chakraborty C, Sharma AR, Sharma G, Doss CGP, Lee SS: Therapeutic miRNA and siRNA: Moving from Bench to Clinic as Next Generation Medicine. Mol Ther Nucleic Acids 2017, 8: 132-143.
- Devic S: Warburg Effect - a Consequence or the Cause of Carcinogenesis? J Cancer 2016, 7(7): 817-822.
- Solaini G, Sgarbi G, Baracca A: Oxidative phosphorylation in cancer cells. Biochim Biophys Acta 2011, 1807(6): 534-542.
- Wang Q, Ye B, Wang P, Yao F, Zhang C, Yu G: Overview of microRNA-199a Regulation in Cancer. Cancer Manag Res 2019, 11: 10327-10335.
- Hou J, Lin L, Zhou W, Wang Z, Ding G, Dong Q, Qin L, Wu X, Zheng Y, Yang Y, et al: Identification of miRNomes in human liver and hepatocellular carcinoma reveals miR-199a/b-3p as therapeutic target for hepatocellular carcinoma. Cancer Cell 2011, 19(2): 232-243.
- Wang Z, Ma X, Cai Q, Wang X, Yu B, Cai Q, liu B, Zhu Z, Li C: MiR-199a-3p promotes gastric cancer progression by targeting ZHX1. FEBS Lett 2014, 588(23): 4504-4512.
- Yang X, Zheng Y, Tan J, Tian R, Shen P, Cai W, Liao H: MiR-199a-5p-HIF-1α-STAT3 Positive Feedback Loop Contributes to the Progression of Non-Small Cell Lung Cancer. Front Cell Dev Biol 2020, 8: 620615.
- Ye H, Pang L, Wu Q, Zhu Y, Guo C, Deng Y, Zheng X: A critical role of mir-199a in the cell biological behaviors of colorectal cancer. Diagn Pathol 2015, 10: 65.
- Yang X, Ma L, Wei R, Ye T, Zhou J, Wen M, Men R, Aqeilan RI, Peng Y, Yang L: Twist1-induced miR-199a-3p promotes liver fibrosis by suppressing caveolin-2 and activating TGF-β pathway. Signal Transduct Target Ther 2020, 5(1): 75.
- Kim W, Kyung Lee E: Post-transcriptional regulation in metabolic diseases. RNA Biol 2012, 9(6): 772-780.
- Jewer M, Findlay SD, Postovit LM: Post-transcriptional regulation in cancer progression : Microenvironmental control of alternative splicing and translation. J Cell Commun Signal 2012, 6(4): 233-248.
- Li L, Wang Y, An L, Kong X, Huang T: A network-based method using a random walk with restart algorithm and screening tests to identify novel genes associated with Menière's disease. PLoS One 2017, 12(8): e0182592.
- Nolfi-Donegan D, Braganza A, Shiva S: Mitochondrial electron transport chain: Oxidative phosphorylation, oxidant production, and methods of measurement. Redox Biol 2020, 37: 101674.
- Urra FA, Weiss-López B, Araya-Maturana R: Determinants of Anti-Cancer Effect of Mitochondrial Electron Transport Chain Inhibitors: Bioenergetic Profile and Metabolic Flexibility of Cancer Cells. Curr Pharm Des 2016, 22(39): 5998-6008.
- Shin D, Lee J, You JH, Kim D, Roh JL: Dihydrolipoamide dehydrogenase regulates cystine deprivation-induced ferroptosis in head and neck cancer. Redox Biol 2020, 30: 101418.
- Han Q, Yan P, Song R, Liu F, Tian Q: HOXC13-driven TIMM13 overexpression promotes osteosarcoma cell growth. Cell Death Dis 2023, 14(7): 398.
- Zou X, Tang XY, Qu ZY, Sun ZW, Ji CF, Li YJ, Guo SD: Targeting the PDGF/PDGFR signaling pathway for cancer therapy: A review. Int J Biol Macromol 2022, 202: 539-557.
- Farooqi AA, Siddik ZH: Platelet-derived growth factor (PDGF) signalling in cancer: rapidly emerging signalling landscape. Cell Biochem Funct 2015, 33(5): 257-265.
- Chen J, Yuan W, Wu L, Tang Q, Xia Q, Ji J, Liu Z, Ma Z, Zhou Z, Cheng Y, et al: PDGF-D promotes cell growth, aggressiveness, angiogenesis and EMT transformation of colorectal cancer by activation of Notch1/Twist1 pathway. Oncotarget 2017, 8(6): 9961-9973.
- Yeeravalli R, Kaushik K, Das A: TWIST1-mediated transcriptional activation of PDGFRβ in breast cancer stem cells promotes tumorigenesis and metastasis. Biochim Biophys Acta Mol Basis Dis 2021, 1867(7): 166141.
- Eckert MA, Lwin TM, Chang AT, Kim J, Danis E, Ohno-Machado L, Yang J: Twist1-induced invadopodia formation promotes tumor metastasis. Cancer Cell 2011, 19(3): 372-386.
- Fauziya, Gupta A, Nadaf A, Ahmad S, Hasan N, Imran M, Sahebkar A, Jain GK, Kesharwani P, Ahmad FJ: Dasatinib: a potential tyrosine kinase inhibitor to fight against multiple cancer malignancies. Med Oncol 2023, 40(6): 173.
Asia-Pacific Journal of Surgical & Experimental Pathology
ISSN 2977-5817 (Online)
Copyright © Asia Pac J Surg Exp & Pathol. This
work is licensed under a Creative Commons AttributionNonCommercial-No Derivatives 4.0 International (CC BY-NC-ND 4.0)
License.